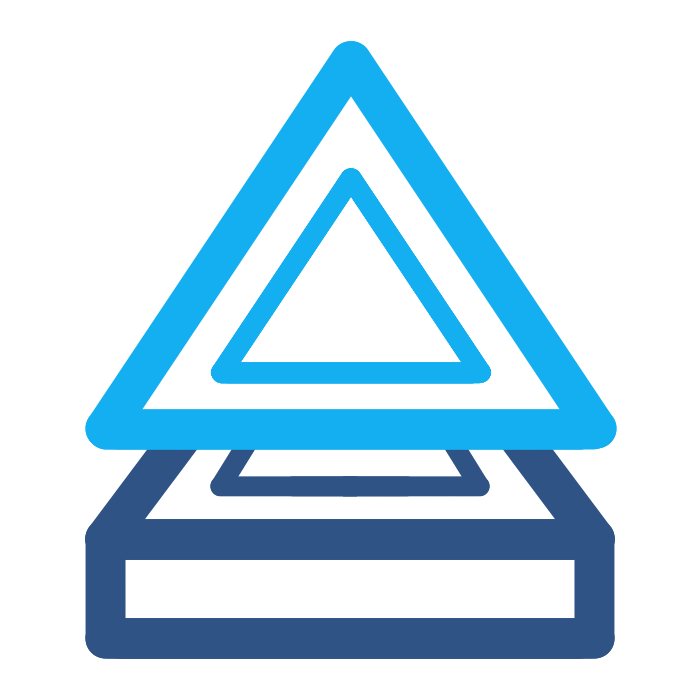
Foundation models in artificial intelligence are large-scale, pre-trained models that serve as a base for building more specific, fine-tuned models. They are often created using deep learning techniques, such as neural networks, and are trained on massive amounts of data from diverse sources.
The term “foundation models” gained prominence with the advent of models like OpenAI’s GPT-3 and its successors. These models demonstrated impressive capabilities across a wide range of tasks, such as language understanding, translation, summarization, and even code generation, with relatively little fine-tuning.
Foundation models have several key characteristics:
- Scale: They involve large architectures, often with billions of parameters, and require significant computational resources to train.
- Transfer learning: They are designed to leverage the knowledge gained during pre-training to be fine-tuned for a variety of specific tasks. This makes them highly adaptable and efficient.
- Multi-domain learning: Foundation models can learn from diverse data sources, which allows them to acquire a broad range of skills and knowledge.
- Few-shot or zero-shot learning: They can often perform well on general-purpose tasks with minimal training examples or even without any additional training, by leveraging the general knowledge acquired during pre-training.
Want to stay in the loop?
Join Jaxon’s newsletter!
Despite their remarkable capabilities, foundation models also come with challenges and risks, such as biases present in the training data, misuse of the technology, and environmental impact of the high energy consumption required for training.
Nonetheless, these foundation models serve as a great starting point to create custom machine learning models.